What are Data Completeness metrics?
Identifying the optimal Data Completeness metrics can be challenging, especially when everyday tasks consume your time. To help you, we've assembled a list of examples to ignite your creativity.
Copy these examples into your preferred app, or you can also use Tability to keep yourself accountable.
Find Data Completeness metrics with AI
While we have some examples available, it's likely that you'll have specific scenarios that aren't covered here. You can use our free AI metrics generator below to generate your own strategies.
Examples of Data Completeness metrics and KPIs
Metrics for Data Selection and Rule Development
Tracking your Data Completeness metrics
Having a plan is one thing, sticking to it is another.
Setting good strategies is only the first challenge. The hard part is to avoid distractions and make sure that you commit to the plan. A simple weekly ritual will greatly increase the chances of success.
A tool like Tability can also help you by combining AI and goal-setting to keep you on track.
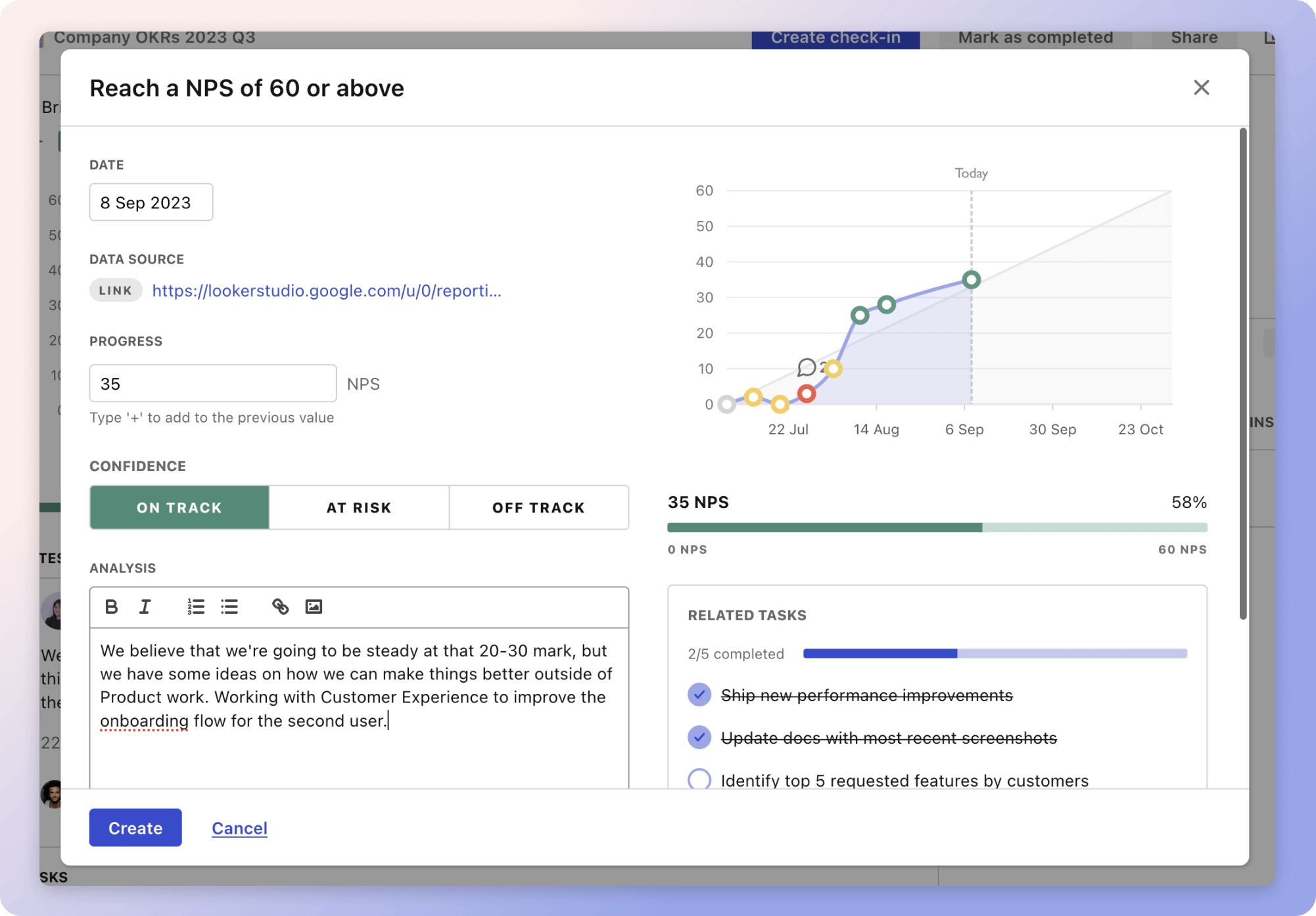
More metrics recently published
We have more examples to help you below.
The best metrics for Accuracy in Multiple Warehouses
The best metrics for Data Selection and Rule Development
The best metrics for Employee Increment Program
The best metrics for Reduce CLABSI in ICU
The best metrics for Profit increase strategies
The best metrics for Speed and Security Analysis
Planning resources
OKRs are a great way to translate strategies into measurable goals. Here are a list of resources to help you adopt the OKR framework:
- To learn: What are OKRs? The complete 2024 guide
- Blog posts: ODT Blog
- Success metrics: KPIs examples