What are Data Science metrics?
Identifying the optimal Data Science metrics can be challenging, especially when everyday tasks consume your time. To help you, we've assembled a list of examples to ignite your creativity.
Copy these examples into your preferred app, or you can also use Tability to keep yourself accountable.
Find Data Science metrics with AI
While we have some examples available, it's likely that you'll have specific scenarios that aren't covered here. You can use our free AI metrics generator below to generate your own strategies.
Examples of Data Science metrics and KPIs
Metrics for Evaluating a Sourcing Model
Tracking your Data Science metrics
Having a plan is one thing, sticking to it is another.
Don't fall into the set-and-forget trap. It is important to adopt a weekly check-in process to keep your strategy agile – otherwise this is nothing more than a reporting exercise.
A tool like Tability can also help you by combining AI and goal-setting to keep you on track.
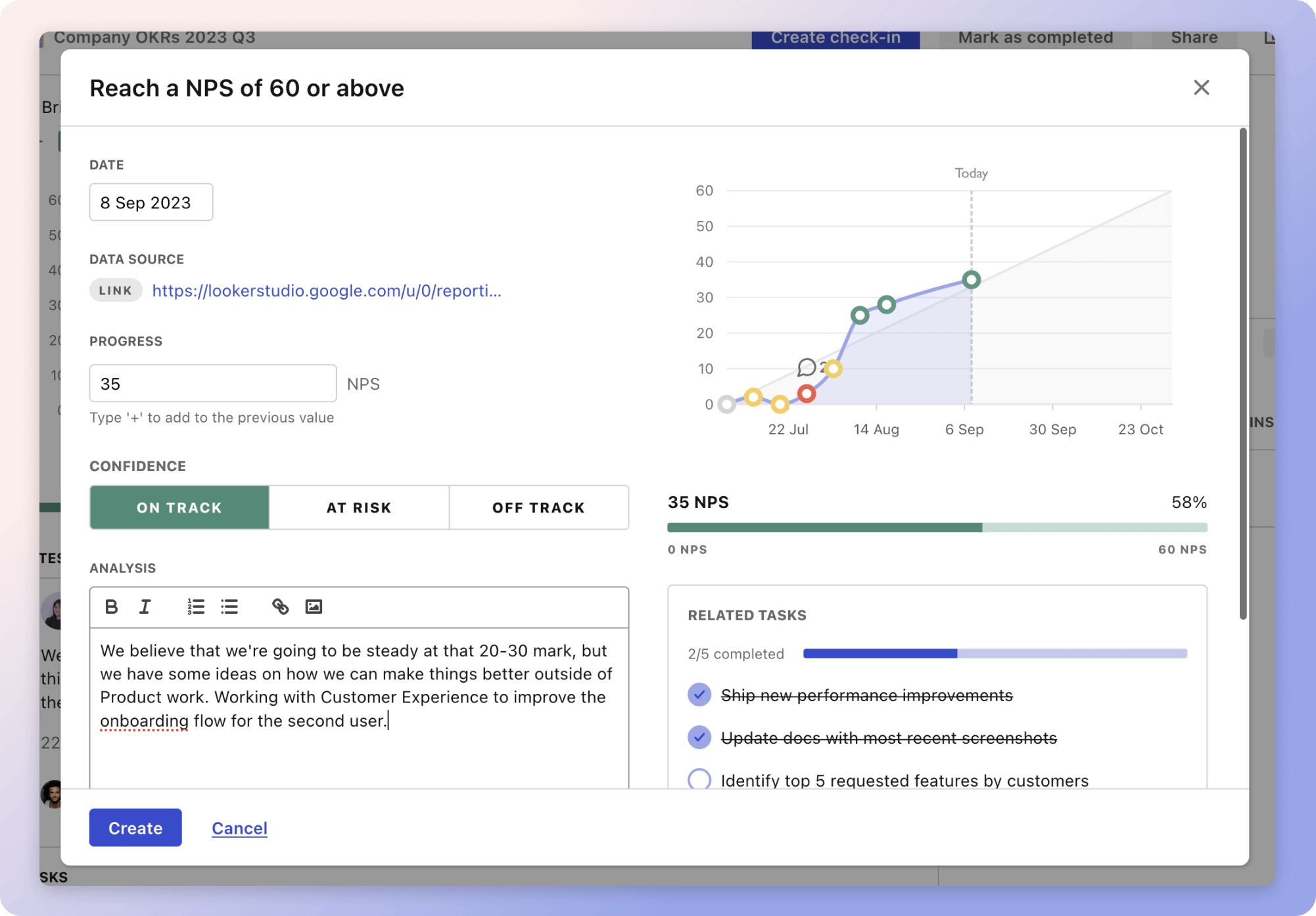
More metrics recently published
We have more examples to help you below.
The best metrics for Establish design standards
The best metrics for Coverage and Pricing Strategy
The best metrics for UX Performance and Quality
The best metrics for Container Movement Tracking
The best metrics for Addressing UX Issues
The best metrics for Automation in Business Units
Planning resources
OKRs are a great way to translate strategies into measurable goals. Here are a list of resources to help you adopt the OKR framework:
- To learn: What are OKRs? The complete 2024 guide
- Blog posts: ODT Blog
- Success metrics: KPIs examples